Harnessing the Power of Machine Learning in Workplace Management
“Machine learning is revolutionizing how organizations manage their workplaces, from optimizing workflows and enhancing knowledge management to improving security and enabling predictive analytics. This article explores practical applications of machine learning technologies that HR managers, IT professionals, and facility managers can implement to create more efficient, responsive, and intelligent workplace environments. ”

Harnessing the Power of Machine Learning in Workplace Management
In today's rapidly evolving business landscape, organizations are constantly seeking innovative ways to enhance efficiency, improve decision-making, and create more responsive workplace environments. Machine learning, a subset of artificial intelligence, has emerged as a transformative technology that's reshaping how we approach workplace management across multiple dimensions.
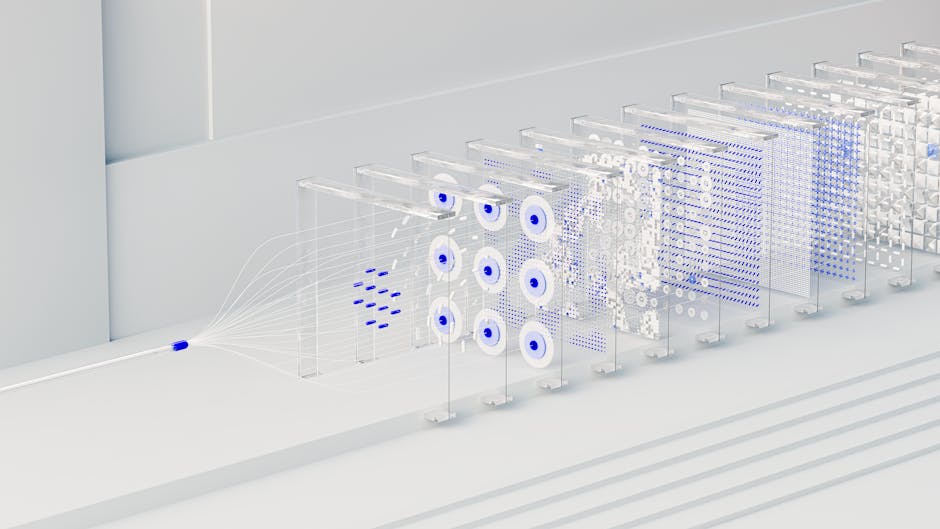
Understanding Machine Learning in the Workplace Context
Machine learning is a branch of artificial intelligence focused on enabling computers to learn from data and improve their performance without explicit programming. Unlike traditional software that follows pre-defined rules, machine learning systems can identify patterns, make predictions, and continuously refine their algorithms as they process more information.
For workplace management professionals—whether in HR, IT, facilities management, or operations—machine learning offers powerful tools to address complex challenges:
- Data-driven decision making: Converting vast amounts of workplace data into actionable insights
- Process automation: Reducing manual tasks and streamlining workflows
- Predictive capabilities: Anticipating needs and challenges before they arise
- Personalization: Tailoring workplace experiences to individual preferences and requirements
The applications of machine learning in workplace management span numerous domains, from optimizing space utilization to enhancing security protocols and improving employee experiences.
Optimizing Workflows with Machine Learning
One of the most immediate applications of machine learning in workplace management is workflow optimization. Traditional workflow management often relies on static processes that may not adapt well to changing conditions or unique situations.
Machine learning transforms this approach by:
- Identifying inefficiencies: Analyzing process data to detect bottlenecks, redundancies, and opportunities for improvement
- Automating routine tasks: Using intelligent algorithms to handle repetitive processes, freeing up human resources for more complex work
- Recommending improvements: Suggesting process modifications based on historical performance data
- Adapting to changing conditions: Continuously learning from new data to refine workflows over time
For example, a machine learning system might analyze how meeting rooms are utilized across an organization, then automatically suggest optimal scheduling patterns that reduce conflicts and maximize space efficiency. Similarly, it could examine document approval processes to identify unnecessary steps or common delay points, then recommend streamlined alternatives.
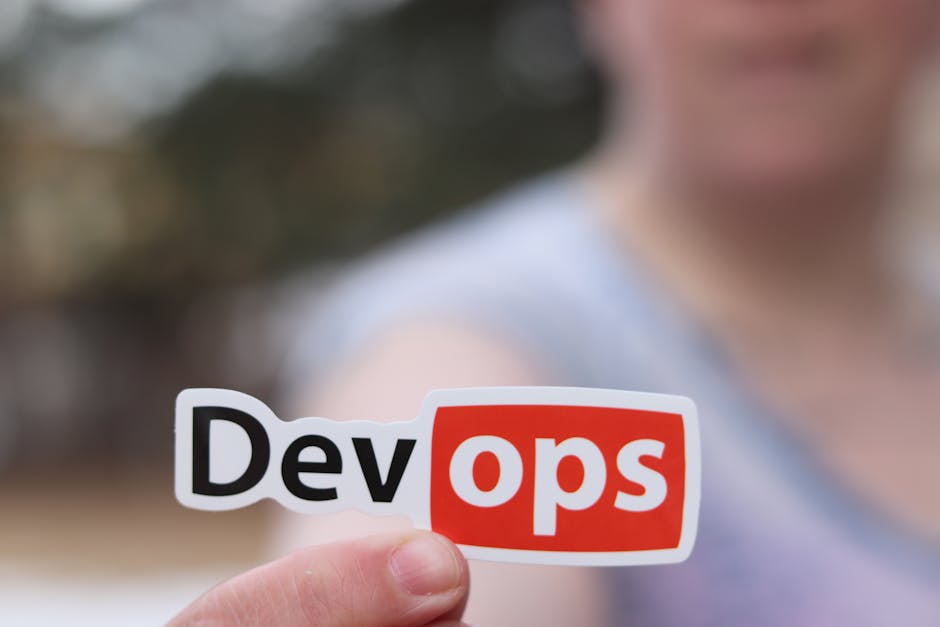
Enhancing Knowledge Management Through AI
Knowledge management represents another area where machine learning is making significant impacts. As organizations accumulate vast repositories of information, finding relevant knowledge quickly becomes increasingly challenging.
Machine learning addresses this challenge through:
- Intelligent search capabilities: Going beyond keywords to understand the context and intent behind queries
- Automated categorization: Organizing information logically without manual tagging
- Content recommendations: Suggesting relevant resources based on user behavior and needs
- Knowledge gap identification: Highlighting areas where organizational knowledge is incomplete
For instance, a machine learning-powered knowledge management system might analyze which documentation new employees access most frequently, then automatically prioritize those resources during onboarding. Or it might notice patterns in support tickets to identify topics requiring better documentation, then suggest content improvements.
Predictive Analytics for Facility Management
Facility managers face the ongoing challenge of maintaining optimal workplace environments while controlling costs. Predictive analytics, powered by machine learning, offers powerful new approaches to these challenges.
Key applications include:
Space Utilization Forecasting
Machine learning algorithms can analyze historical occupancy data, meeting patterns, and seasonal trends to predict future space needs with remarkable accuracy. This enables organizations to:
- Optimize room configurations based on actual usage patterns
- Identify underutilized spaces that could be repurposed
- Plan expansions or consolidations with greater confidence
- Implement dynamic space allocation based on predicted needs
Preventive Maintenance
Rather than waiting for equipment to fail or relying on fixed maintenance schedules, machine learning enables predictive maintenance approaches:
- Analyzing sensor data to detect early warning signs of equipment issues
- Predicting when maintenance will be needed based on usage patterns
- Optimizing maintenance scheduling to minimize disruptions
- Extending equipment lifespan through timely interventions
Energy Optimization
Machine learning systems can significantly reduce energy consumption by:
- Learning occupancy patterns to adjust HVAC and lighting systems automatically
- Identifying anomalous energy usage that might indicate equipment problems
- Optimizing energy consumption based on weather forecasts and building usage
- Recommending energy-saving modifications based on usage analysis
Enhancing Security and Compliance
Information security and regulatory compliance represent critical concerns for workplace managers. Machine learning provides powerful tools for addressing these challenges proactively rather than reactively.
Threat Detection and Response
Traditional security approaches often rely on known threat signatures, making them vulnerable to new attack methods. Machine learning enhances threat detection by:
- Establishing baseline patterns of normal network behavior
- Identifying anomalous activities that might indicate security threats
- Continuously adapting to new threat patterns
- Automating initial response actions to contain potential breaches
Data Privacy Protection
As data privacy regulations become increasingly stringent, machine learning helps organizations maintain compliance through:
- Automated identification of sensitive data across systems
- Intelligent redaction and anonymization of personal information
- Monitoring for unauthorized access or unusual data movement
- Flagging potential compliance issues before they become violations
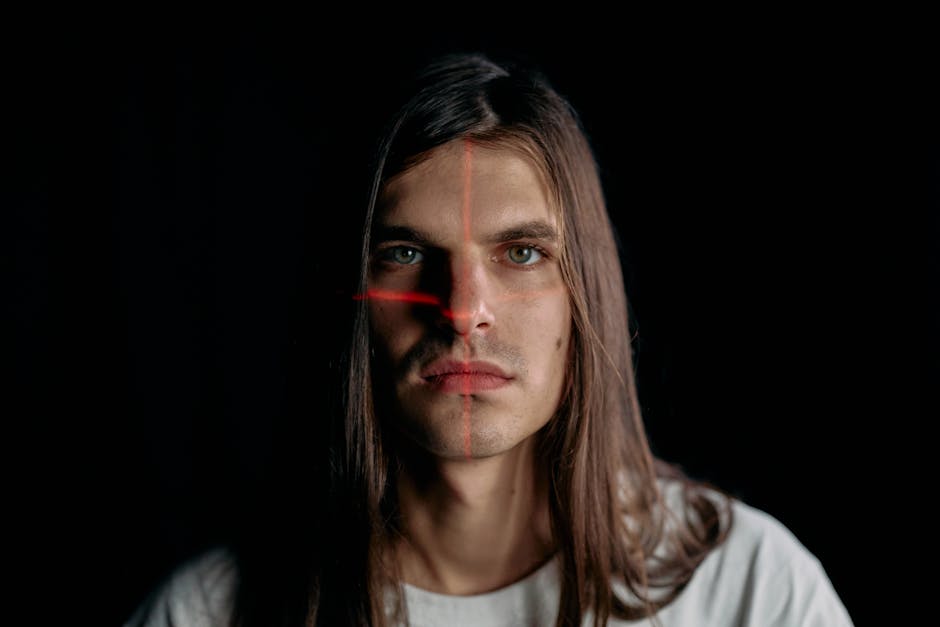
Incident Response Automation
When security incidents do occur, incident response time is critical. Machine learning accelerates response by:
- Automatically classifying incidents by type and severity
- Recommending appropriate response actions based on incident characteristics
- Learning from past incidents to improve future responses
- Identifying patterns that might indicate coordinated attacks
Improving Employee Experience and Engagement
Beyond operational efficiencies, machine learning can significantly enhance how employees experience their workplace environment.
Personalized Workplace Experiences
Just as consumer applications use machine learning to personalize experiences, workplace systems can adapt to individual preferences:
- Automatically adjusting environmental settings based on personal preferences
- Customizing information dashboards to highlight relevant content
- Recommending relevant learning resources based on career goals and work patterns
- Adapting communication channels to individual preferences
Enhanced Collaboration
Machine learning can facilitate more effective collaboration by:
- Recommending optimal team compositions based on skills and past collaboration patterns
- Suggesting relevant collaboration tools for specific project types
- Identifying potential mentorship or knowledge-sharing opportunities
- Optimizing meeting schedules to maximize productivity
Proactive Wellbeing Support
Employee wellbeing has become a central concern for workplace managers. Machine learning offers new approaches to supporting wellbeing:
- Detecting patterns that might indicate burnout risk
- Recommending breaks or wellness activities based on work patterns
- Optimizing work schedules to reduce stress and maximize productivity
- Personalizing wellbeing resources to individual needs and preferences
Implementation Considerations for Workplace Managers
While the potential benefits of machine learning are substantial, successful implementation requires careful planning and consideration of several key factors.
Data Quality and Availability
Machine learning systems are only as good as the data they learn from. Organizations should:
- Audit existing data sources for completeness and accuracy
- Implement data governance processes to maintain quality
- Consider privacy and ethical implications of data collection
- Develop strategies for addressing data gaps
Change Management
Introducing machine learning systems often requires significant changes to established processes. Effective change management includes:
- Clearly communicating the purpose and benefits of new systems
- Providing adequate training and support during transitions
- Starting with pilot projects to demonstrate value
- Gathering and responding to user feedback
Ethical Considerations
Machine learning implementations raise important ethical questions that organizations must address:
- Ensuring algorithms don't perpetuate or amplify existing biases
- Maintaining appropriate human oversight of automated decisions
- Protecting individual privacy while leveraging collective data
- Being transparent about how machine learning systems make decisions
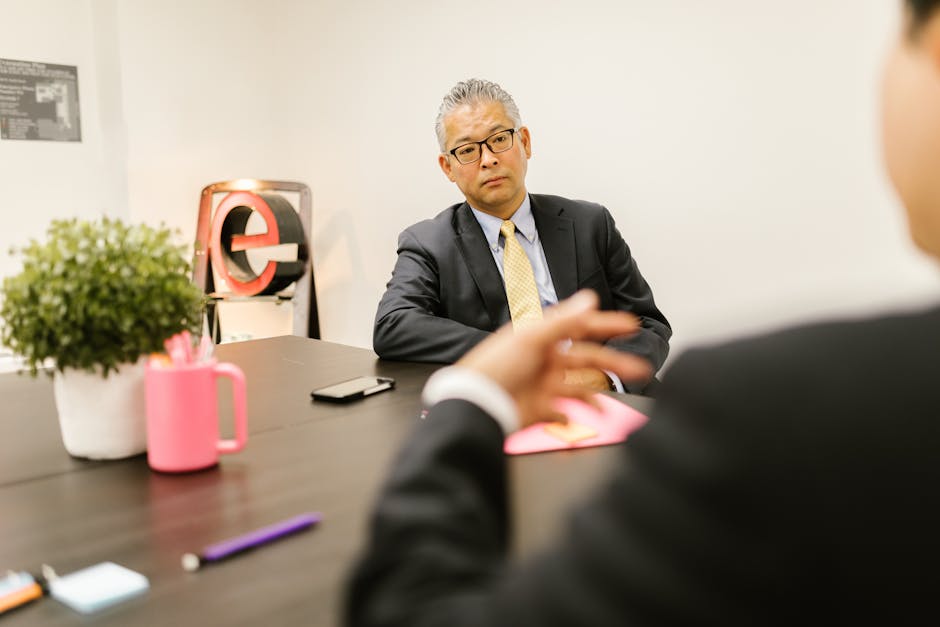
The Future of Machine Learning in Workplace Management
As machine learning technologies continue to evolve, we can anticipate several emerging trends that will further transform workplace management:
Integration with Other Advanced Technologies
Machine learning will increasingly work in concert with other technologies:
- Internet of Things (IoT): Expanding the range and granularity of workplace data
- Augmented Reality: Creating new interfaces for interacting with intelligent systems
- Voice Interfaces: Enabling more natural interactions with workplace systems
- Robotics: Extending automation beyond digital processes to physical tasks
Increasingly Autonomous Systems
While current machine learning systems typically augment human decision-making, future systems will likely take on more autonomous functions:
- Automatically reconfiguring workspaces based on changing needs
- Proactively addressing potential issues before they're apparent to human managers
- Optimizing complex systems with minimal human intervention
- Continuously adapting to changing conditions without explicit reprogramming
Democratization of Machine Learning Tools
As machine learning tools become more accessible, we'll likely see:
- More workplace managers implementing machine learning without specialized expertise
- Greater customization of machine learning applications to specific organizational needs
- Faster adoption of machine learning across organizations of all sizes
- More innovation in how machine learning is applied to workplace challenges
Conclusion: Preparing for the Machine Learning-Enhanced Workplace
For HR managers, IT professionals, facility managers, and other workplace leaders, machine learning represents both an opportunity and a challenge. The potential benefits in terms of efficiency, insight, and enhanced experiences are substantial, but realizing these benefits requires thoughtful implementation and ongoing adaptation.
Organizations that approach machine learning strategically—focusing on clear business objectives, ensuring data quality, addressing ethical considerations, and managing change effectively—will be well-positioned to create more intelligent, responsive, and human-centered workplaces.
The future workplace will not be one where machines replace human judgment, but rather one where machine learning amplifies human capabilities, automates routine tasks, and provides insights that would otherwise remain hidden. By understanding and embracing these possibilities, workplace management professionals can lead their organizations toward more efficient, effective, and engaging work environments.